Cutting Through the Fog of GenAI Pricing
It’s been a little over a year since ChatGPT marked a watershed moment for GenAI and became the most rapidly adopted application of all time. Hardly a day goes by without a forecast of the potential for GenAI to transform how companies will research and develop products, interact with customers, or make their entire operations more efficient.
Talk about value creation!
Any conversation about value creation should lead directly to a conversation about pricing, and that usually causes a stampede to the “money” question: how much should a company charge for its GenAI applications?
Whether that is the million-dollar or billion-dollar question depends not so much on the number the company comes up with as it does on how the company figures out the answer. With so much at stake in a market still in its infancy, the answer to the “how much?” should be the last thing on a business leader’s mind at the moment. The missing piece in such a nebulous yet growing market is a lens to see through the fog and develop a pricing strategy.
How to gather your pricing thoughts
There is general consensus that there is no one-size-fits-all strategy for pricing a GenAI application. But there also aren’t any standard off‐the‐shelf pricing strategies that companies can quickly cut and paste onto their own situation. This has led to a Wild West of experimentation, as companies test all sorts of pricing models, metrics, lineups, and other designs. Most are proving to be sub-optimal so far, because it is hard to get a handle on the fundamental pricing dynamics in these complex markets.
Costs are changing quickly, as we described in this article in The Wall Street Journal. Many of these applications require massive computing power and massive intelligence. Competitors are entering the market all the time, and the ecosystem is constantly evolving as customers start to imagine the possibilities and do their own experimentation.
With these basic strategic inputs all in flux, the Strategic Pricing Hexagon provides an anchor. To apply it, we first start by grouping the emerging GenAI applications into three categories, with each one building on the next.
- Foundational applications are models pre-trained on vast amounts of data. They show generative capabilities and serve as backbones for the entire GenAI ecosystem. Examples include OpenAI.
- GenAI-powered applications are customer-facing applications built on top of foundational models. They have an easy-to-use user interface and can provide fine-tuned applications using customer data. Microsoft 365 Copilot is an example.
- Automation workflows are built on GenAI-powered applications with the goal of automating workflows end-to-end for a given task or function. These capabilities are still emerging, but ideally they can turbocharge value creation, because they autonomously execute tasks with little or no human supervision.
Each of these groups fit to specific games within the Hex, as the figure below shows.
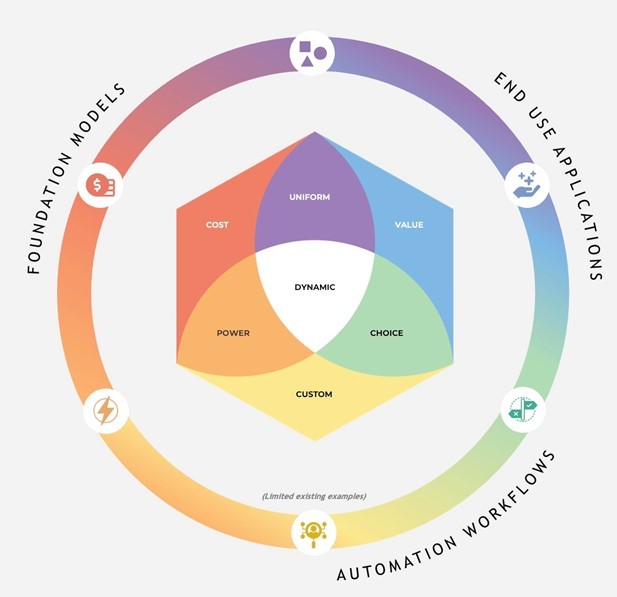
There are no one-to-one matches, however, between a type of offering and a game. In each case, leaders need to decide which game to play, and more importantly, the pricing models to use.
The opportunities and risks of choosing a pricing model
The most common models for GenAI applications are consumption, subscription, and outcome-based. Players of the games on the left and upper sides of the Hex can start with consumption models using a cost-plus approach. Tokens, the main unit of consumption, are basically proxies for costs, and marginal costs are high for some GenAI models. While some companies may use this as a rationale to charge higher prices in the short term, the reality is that costs for a given model performance will decline in a “Moore’s Law” fashion.
It will be hard for enterprise customers to predict and manage their spend if tokens are costs and consumption varies massively. This will create pressure on companies to shift to a more predictable pricing basis such as users or outcomes.
Players of the games on the right and lower sides of the Hex are a better fit for subscription or outcome-based models. When Microsoft revealed its vision for its Copilot “digital companion” it affirmed price points of $30 per month for enterprise customers. This kind of model is easy to understand for both parties, but the history of flat rates in early stages of other markets – such as internet usage and mobile telephony – shows that unit economics can quickly become challenging if usage varies significantly across customers.
Another risk comes from equating output to outcome, which can lead to the assumption that consumption pricing aligns well with value delivered. That would make consumption the “end-all” pricing model for GenAI. But this simple example for an automated customer service chatbot shows why this confusion is risky. A consumption-based model works when the pricing metric is the number of interactions the chatbot has with customers. An outcome-based model works when the pricing metric is, for example, the number of cases closed that are rated “satisfied” by a customer. The latter group may be much smaller in volume, but much more valuable for the company.
Will GenAI replace humans?
Believe it or not, the choices that companies make about pricing can also shed light on that burning question. Look at the pricing metrics and you’ll learn the fate of humanity. Models that have a subscription per user are about making humans more productive, not about eliminating their jobs. Alternatively, models whose pricing metric is based on outcomes or tasks are inherently replacing humans.
What will the future hold?
We expect a few trends to take hold as this market evolves and the fog clears. The costs of foundation models will soon reach an optimal level at which they no longer have meaningful impact on the topline economics of GenAI apps. As competition intensifies, more GenAI applications will continue to transition to the Choice and Custom Games. This will make pricing less transparent and more complex.
New products, such as automation workflows, and different pricing schemes, such as outcome-based pricing, will further reshape the GenAI market landscape. We expect outcome-based pricing to take hold eventually as GenAI market matures, as it aligns better with the value delivered for end-to-end solutions.
Strategic pricing decisions that companies make right now are fundamental, because they will affect who benefits from GenAI, how fast adoption comes, how much money companies can re-invest into improvements and competitive advantages, and ultimately, how lucrative this business is in the long run. Making those decisions starts with putting the right thought process in place. We will elaborate on these ideas and provide more specific guidance in an upcoming article on BCG.com.
View this edition of The Game Changer Newsletter on LinkedIn